Explore Our Insights
-
Efficiency, Interoperability and Trust: Digital Health Shifts to Meet Providers’ Needs in 2025
-
Business Services: 2025 Tariff Impact on Transportation & Logistics
-
Technology: Aligning Sales and Marketing with Commercial Sales
-
Life Sciences: Driving Growth Through Commercial Blueprint Design
-
Technology: The Go-to-Market Planning Process – A Comprehensive Guide
-
Packaging: Optimizing the Coverage Model for Sales Success
-
Private Equity Trends: AI, Talent Strategies and Value Creation Insights
-
Celebrating 40 Years of Service
-
Technology: RevOps – The Missing Link Between AI Models & Business Value
-
Life Sciences: Adapting to Market Pressures and Patient-Centric Demands in Biotech and Pharma
-
Business Services: Navigating Go-to-Market Challenges for Professional Services Leaders
-
Technology: GTM Strategies for Revenue Growth and Customer Acquisition
-
Tariffs: Revenue Leadership in Times of Disruption
-
Marketing Operations: Optimizing Upstream-Downstream Enablement for Growth
-
Cross-Industry: MQL to SQL – Best Practices for Optimizing Lead Flow
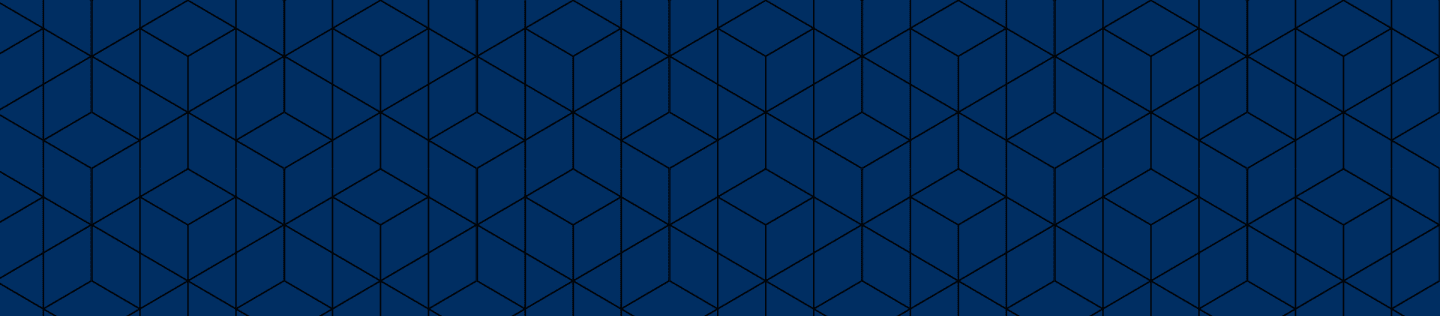
Alexander Group has helped thousands of clients.
Our expert leaders assess, align, design and implement powerful sale compensation programs. Alexander Group has helped organizations realize the full benefits of effective sales compensation programs to attract, retain and reward best-in-class sales talent to profitably grow the business. We can help with all elements of your sales compensation program. Contact us today.