Embrace Disruption: Five AI Use Cases to Support Your 2024 Revenue Growth Plan
The growing popularity of generative AI has opened up a wide variety of potential use cases and opportunities, leaving many executives wondering where should they be spending time to focus on the greatest impact.
Alexander Group Director Davis Giedt and Andrew Morales, senior director of GTM Strategy and Operations at Captivate IQ, joined Mark Schopmeyer from Captivate IQ to share a practitioner’s lens on what is being used today in AI.
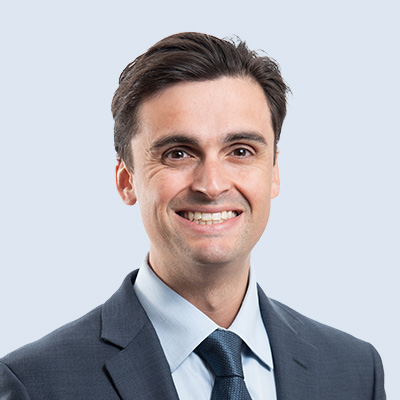
Mark Schopmeyer : Welcome to the webinar today, Embracing Disruption. Five AI use cases support the 2024 Growth revenue growth plan. The growing popularity of Gen AI has opened up a lot of variety of potential use cases and opportunities, leaving many folks like yourselves wondering where should they be spending time to focus with the greatest impact. Today I’m joined by the Alexander Group’s Davis Giedt, who wrote a post on the topic, and from our own Andrew Morales, senior director of GTM Strategy and Operations at Captivate IQ, who will be sharing more of a practitioner’s lens on what is being used today. Our goal is to make sure that there’s much more of this back-and-forth conversation, from the research to practitioners, so you all get the best insight.
Before jumping in, there’s a couple of housekeeping items. Well, first we have this today’s session will be recorded and we’ll share that shortly after this email. So if you miss any part of it, you’ll be able to catch the full length of it. Please use the chat for us or with this, if you want to engage a little bit more or react to some of the topics that we’re sharing today, we’ll also save some, uh, Q&A at the end. So please, I encourage everyone to add their questions to make sure that you’re getting what you want and some of the insights as you think about these big topics coming into 2024. And lastly, for more information on future sessions and other resources like this, please follow us on LinkedIn and please follow our Alexander Group on LinkedIn. With that said, Davis and Andrew, would you mind giving a brief background on yourselves?
Davis Giedt: Yeah, sure. Thanks a lot, Mark, for having us here. I’m Davis Giedt, director of the analytics and research practice at the Alexander Group. I’ve been with the company for about 11 years. We’re a consulting firm. We do go-to-market strategy work for companies across sort of the spectrum. Anyone that deals with a larger or mid-size business-to-business go-to-market operation. And we also do quite a bit of research associated with that. Uh, and we’re focused on the AI topic throughout 2024, understanding how AI impacts commercial models and impacts commercial jobs in particular.
Andrew Morales: Awesome. I’m Andrew Morales. I’ve been at Captivate now for just about three months. I’ve spent my entire career in go-to-market. I got my start following UPS trucks at Fedex. That’s how I source my leads as a sales rep and have been on the operations side for for a period of time now. I am a father, husband, son, and I am passionate about all things forecasting. So if anybody on the call wants to chat about forecasting, you got my LinkedIn info and feel free to reach out. I’m excited about our chat today.
Mark Schopmeyer : Awesome, great. And my background myself co-founder co-CEO of captivate IQ. I’ve been spending a lot more of my time around commissions in a prior life, and I’m very excited to be talking about this topic with you both today, so we’ll let this set the stage. Davis, you published on this topic recently. Would you mind running through a quick overview of what you learned on the five applicable use cases of AI within GTM?
Davis Giedt: Yeah, absolutely. I think it’s a good place to start for today. What we found is that all companies just take a step back. All companies operating at scale have had to work through at least one significant market disruption in our lifetime. And we treat AI not unlike other market disruptions that we’ve seen. Um, you know, for example, the 2008 financial crisis going back a few years, um, and then some more sort of positive ones, the rise of e-commerce and data science and business-to-business interactions. About ten years ago, it became very popular. And then, you know, not to end on a sour note, but Covid in 2020 was another disruption that organizations had to weather through. We think of AI as a disruption, but it’s a little bit different. It’s a it’s a tool that enables people and process and productivity. And we see great things for go-to-market organizations as it relates to AI. One thing to start with, at the beginning of 2020, for the universe of possible AI investments is really intimidating for a lot of leaders, particularly in the go-to-market space. And it’s hard to know where to begin.
And so that’s one of the reasons why we published this article around five use cases that could support growth. This is based on sort of five more tangible use cases that we’re seeing in the market that organizations are beginning to invest in. It’s still early days, but organizations are starting to focus on these. So I’ll run through each one of them kind of briefly, and then I’ll hand it back to Mark, and we can talk about what we’re going to focus on today in greater detail.
The first one centers around sort of creating an AI-enabled lead generation engine and this is a pretty popular use case that goes across marketing and sales. We’ve seen a lot of organizations use AI to increase the quantity and the quality of leads. It also helps to improve conversion rates and in some cases, more efficiently target certain types of buyers, like large enterprise buyers or small sort of SMB type of buyers.
The second one which I believe we’re going to focus on today is maximizing engage selling time. So maximizing high-value time for sales reps, in particular using AI sales assistants. Our research shows that really a minority of a sales reps time on average, is focused on what we consider high-value, customer-facing engage selling activities. And we’ve seen some early indications that using AI sales assistants could actually offer a seller more engaged selling time by way of taking away some of their administrative tasks like updating CRM. Doing account planning, transcribing notes, sort of staying organized, staying intelligent on what buyers are doing in particular.
The third one that we see is around sort of leveraging AI for real-time coaching, whether it’s delivered by the tool itself or delivered by management. So this is a way this is using AI to sort of enable not only seller but also management. So we’ve seen AI tools that analyze transcripts. They’ll actually in a in a call, they’ll actually analyze body language and tone to help sellers identify what tactics are working with their buyers, what aren’t. We’ve seen tools that proactively coach sellers on tone, so they actually give them real-time input and commentary on on improving their tone to sort of drive towards better outcomes. And then on the management side of things, we’ve seen a lot of AI tools that share summaries of performance and summaries of calls and whatnot with management to allow managers to not only create a library of best practices that they can then deliver to their reps, saying, here’s where I expect you to sort of strive for, but also looking for areas for improvement and ways that reps can sort of improve their talk track as they as they grow in their careers.
The fourth one here is about boosting customer experience through AI-powered service interactions. This one goes pretty wide, but the most common use case we’re seeing in the most developed one is AI. Chatbots that automate a lot of the interactions in a service world. So think of this as sort of your simplified, repetitive customer service interaction. There’s a lot of value to using an AI chatbot that’s loaded with lots of Intel around resolved service cases and different types of buyers over the past. It’s also a really powerful tool for service reps to use, much like a sales assistant to guide them in their interactions and ultimately drive towards a better outcome, better customer experience.
And then the last one, which I think is near and dear to Andrew, as well as a lot of other folks on the phone, on the line today, empowering rev ops with clean, unified and rich data. There’s a whole host of great AI tools out there that we’ve seen in a lot of good use cases on cleaning data, updating CRM systems automatically, allowing for better analysis and replacing of certain types of data with better, higher quality sources, organizing things, driving towards predictive outcomes and ultimately providing better forecast and better visibility to what the organization is doing. So these are really just five use cases to think about there emerging ones that some survey data that we have seen from our sales poll survey that just came out in the January time frame tell it’s telling us that these are kind of five of the top use cases that commercial organizations are focused on, but there are many more. I guess with that, I’ll hand it back to Mark and we can we can start talking about sort of which ones we’re going to focus on today.
Mark Schopmeyer : Great recap. There’s a lot to unpack. Uh, and we’ve heard from the audience that they wanted to spend a little bit more time around three particular use cases for discussion. So we’re going to be covering maximizing engaged selling time with AI sales assistants, boost customer experience with AI powered service interactions, and empower rev ops with clean, unified and enriched data. So let’s start with the first one maximizing engage selling time with AI sales assistants. Davis, maybe you can share a little bit. Why should this matter for business leaders and sellers? And what did you learn in your research?
Davis Giedt: Yeah, yeah, it’s a great question Mark. Um, you know what we’ve found for years we’ve been doing sales time studies for many, many years with our clients. What we do is we go in and we ask, you know, hundreds of sales reps or certain organizations, how do they spend their time across ten different categories, whether it’s pre-sales activities, so prospecting, account planning, etc.. Uh, uh, engage selling activities which are actually, uh, uh, interacting with the customer around sort of closing activities and, and persuasion activities, sales completion. So whether that’s order entry or, uh, order facilitation and then what we consider sales facilitation or admin activity. So admin and reporting uh non-productive travel time. And what we found is that the average sales rep only spends about 25%. So about a quarter of their time in engage selling. So there’s a lot of time left on the table if you will. Um, that could potentially be, uh, sort of solved for by taking away from other activities that reps have to focus on. So one of the sort of lowest hanging fruit there is minimizing routine administrative tasks. So offering sort of more selling time to sellers. And we’ve seen a lot of ways that I can support in that.
Mark Schopmeyer : It’s awesome. Andrew, I’d love to get your reactions to HGH Do you mind sharing? How do you you know, you’ve been at several businesses, both big and large or big and small, clearly one that actually focused on sales rep productivity. This resonates in a big way. Can you share what tips you are resonating and why?
Andrew Morales: Yeah, absolutely. So all three of the things that Davis outlined pre-sales activities, sales completion, sales facilitation, all of them resonate with me from the same point that from a revops perspective, what we focus on quite a bit is this idea of seller productivity. And for us here at CIQ, in the way that I like to think about it, is it’s a pretty simple equation. It’s expenses over bookings. And how do we impact either side of that equation to try to drive value for the organization? And when I think about AI and augmenting these various activities, my immediate mind goes to sales completion activities. And so how can we continue to augment workflows to give sellers more time back so that they can focus on value activity, value-added activities like spending more time with customers? And if we give them more time to do that back to do that, then we can increase the bottom part of that equation, which is increasing bookings. And I think that number two really does resonate with me. So, sales completion activities because that’s where a lot of the market has been today. It’s the area I’m most comfortable with, having spent some time at outreach previously as well.
But the area I’m most excited about is actually thinking about pre-sales activities. I think we’re going to get into it a little bit later here, but as we think about our engagement models and how we support our customers, there’s a lot of nuance that goes into, you know, pre-sales activities, planning for an organization, how we think about engaging the organization. And there’s a lot more, I would say, business acumen that comes to light there. So I’m really excited about the progression of that part of sales, AI assistance to augment those activities. I think a lot of the people here on the call today have a lot of experience in sales completion activities, and that’s where a lot of the market’s been focused on from tools and platforms today.
Mark Schopmeyer : Very exciting. Davis, can you share a little more about the specific AI and how it can help? You mind sharing what you’re seeing right now?
Davis Giedt: Yeah, there’s a couple more specific use cases within this world that we’re seeing that sort of impact the rep. So one of them is minimizing administrative work with follow-up, suggestions and automatic logging. So, for example, after a rep finishes an outreach step, a call or an email or whatever, an AI tool can suggest a series of additional tasks that a seller can do to get the prospect closer to sale. And those are based on, in part based on, management training, the model, but also in part based on performance. So looking at top-performing reps and what they do and then using that model can use that to ultimately train mid and low-performing reps on what they should be doing.
To get more specific, one thing that is powerful that we’re seeing a lot of organizations get excited about is automatic CRM logging. So again, tracking what’s going on in a call tracking what’s going on in email, any interaction with a customer and automatically updating CRM. One of the biggest pain points that we’ve seen over the years as evident by interviews that we’ve done, comments and surveys, the sales time surveys that I mentioned is just updating CRM and the amount of time it takes to properly update CRM. Oftentimes, it never actually gets done. I mean, a lot of folks are probably not surprised when they hear that. And so the ability to have an AI tool that at least gets you 80% of the way there. Odds are, in today’s day and age, it’s early days, so you’re not going to have an AI tool that’s going to be able to completely and perfectly update every element of CRM. But just getting you farther along the path not only makes the sales rep feel better about it but can save them, in some cases, studies are showing us it’s saving hours per week. So upwards of 2 to 3 hours per week for a given sales rep.
Mark Schopmeyer : You’re preaching to the choir here. I definitely and I’m sure everyone on this call resonates with automating CRM would be a big boost to just getting that data where it needs to so you can start to make more, higher level decisions. So resonates with me. But maybe, Andrew, what does this look like in practice?
Andrew Morales: Yeah. So, I’ve got two pretty tactical examples as Mark, Davis and I were thinking about what we want people to walk away from this call. It’s really how do we put these in practice today? How do we contextualize these things and the things that we’re doing today? And so I got two examples that I want to share that we’re actually doing here, here at CIQ and that I’ve done in former lives as well. But before diving into both of those, I would like to anchor the way that a lot of these examples, the way we think about them from a framework standpoint is and I will say that you’ll see this in Davis’s article if you take the time to read it as well. Is that really thinking about the contextualization of AI as a technology and a tool to help augment workflows is really important. And going back to the framework and adage of people process technology, AI then lends itself to the technology components of it. So as you back into that, we really need to think about the processes and the people that are in place and what are we expecting them to do in defining those things really for us to maximize the outcome that we want to get from leveraging AI in our in our go-to-market workflows. So that’s a framework that we’ll keep kind of coming back to as we talk about these examples.
One of the examples that we’re specifically doing today is really around automating data capture, as Davis had talked about. And so we are in a position in this ties into one of the use cases a little bit around coaching, where we are trying to get really prescriptive around coaching of our team because we’re really expecting to improve win rates from a plan standpoint this year. And we’ve taken the time to outline what are the expectations of people in the roles and what are the processes that they’re supposed to be doing. And part of those processes are to use tools like outreach and gong for engaging with their customers, so that we’ve automated data capture and activity, and we inspect whether or not our team members are using them so that we can validate and get the data that we’re looking for so that our AI tools can bring those insights to the table. So we’ve defined our process that people are supposed to be using outreach and/or Gong to engage with our customers. And so what is the outcome that we’re getting as a result of that? One of the things that outreach offers is sentiment capture of emails and of engagements with our customers. And that sentiment captures, based on the interactions that are being logged in the platform, and then analyzing all that data to return a sentiment. One of the things that we’re trying to understand is, are some of our reps returning better sentiment from our customers in the way that they’re engaging with them and trying to identify what’s working and what’s not working to then replicate that across the team.
Prior to having an AI assistant-like outreach to identify the sentiment of our engagement with our customers, I would have a mutiny on my hands from our sales organization if I asked them to outline the sentiment of all the emails that they received. And so now we’ve gone through and at scale, we’re able to identify the sentiment of our customers, of our prospects, of how they’re engaging with us so that we can define repeatable processes and scale those across the team. And so just by defining the need to be using one of these platforms to engage with your customers to automate the data capture. We’re then getting insights from those AI assistants to help us improve our workflows and drive better productivity and outcomes for our customers, which within our plan for this year is improving our win rates.
Mark Schopmeyer : Yeah, that last part is huge. I know one thing that we’ve always talked about internally is especially in these markets and these changing markets is getting that sentiment and getting it. You know, I think the old way would be having all these different error code or reason code, I should say. But if you can now capture that more systematically versus having reps fill it out, you get more information, more real-time, which then you can adjust your strategy much faster, which is going to be a lot of either revenue generation or time savings opportunities. Pinging some sales reps, ahead of time just to get their perspective and the S&P questions. I’d love to be able to throw in maybe Davis and Andrew, how ready is a lot of the things that we’re talking about today? For prime time, and I think for sellers themselves are at a stage where people can introduce or even adopt their workflow and said another way. I know there’s a lot of change going on in the markets and people. I’m sure the last thing a seller wants to hear is just another workflow change. How do you guys think about that? Are there bumper rails to make this successful or is it still too early?
Davis Giedt: Yeah, yeah, it’s definitely early days. I mean, let’s remind ourselves that LLMs really became popularized just over a year ago. So it hasn’t been that long since ChatGPT hit the market. Openai went public with everything. For that reason, there’s certain things that are still kind of nascent whenever you look at. But let’s break it into two categories.
If you’re looking at machine learning type of AI models, those have been around for 10+ years. And in a lot of cases, they’re pretty sophisticated. So if you’re talking about lead scoring, if you’re talking about helping reps prioritize different accounts and different opportunities based on propensity to buy and whatnot. So rolling out tools like that have been around for a long time and are a little bit more mature in the marketplace. Any tool that involves a large language model is going to be newer. Having said that, there are some that are sort of beginning to be pretty impactful in the marketplace. When you look at automatic CRM, updating was a combination of machine learning and LLM. In some cases, then the ability to generate at least the startings of certain copy, whether it be for a sales rep, in particular, the startings of a reply email offering them suggestions on a nudge type of email, next steps on a particular account plan, whatnot. There’s different applications that are beginning to be more useful. The problem is the context and the ability to train the model over time and make it more powerful and impactful and relevant to the organization. For a low- or mid-performer, getting that sort of productivity boost or getting a nudge in the right direction from an LLM suggestion is probably pretty powerful. For a high-performing sales rep, may not be all that useful today. There might be other applications that are pretty useful.
Then to your second point on changing sales reps, workflow, this is always an issue. Every time you introduce a new tool or new process, people are going to resist it. It’s just the reality of it. In some cases, they’re going to resist it more. I think it has a lot to do with the culture of the sales organization is a big piece. Secondly, sort of how the sales organization operates. If you’re exclusively an enterprise big deal organization with highly tenured sales reps, maybe AI sales assistants are not going to be as impactful for you immediately. If you’re a high-velocity sales organization with less tenured sales reps and the need to do multiple deals per week, per sales rep, you’re probably going to find value in AI sales assistants, the ability to sort of wrangle your organization in the right direction, given they need a lot of coaching, and need a lot of direction to be able to execute their job effectively.
Andrew Morales: Yeah I think I’d like to start kind of where Davis ended talking about the changing to workflow and then back into the prime time question because it is really interesting. And as new technology comes out, it’s it sometimes can be unnerving or unsettling thinking about introducing something new also. I know that we have a number of revops practitioners on the call today, and oftentimes we might be the ones leading the charge to say we should try something new. And so you can feel like your internal identity or position, you’re putting it out there to introduce something new. So thinking about the change management around process change, I think it goes back to people process technology. If we’ve clearly defined the processes that exist and that we’re expecting people to follow, the technology that we’re introducing should be an augmentation to that. And really, that is where we can reduce the cognitive and change load for our field teams is if we’ve clearly articulated this is how this process is supposed to work, and we’ve been coaching and managing the teams to do that. Now, this is how this process is supposed to work with this new tool in place. And these are the augmentations to it. It reduces the cognitive load.
Mark, as you talked about. There’s a lot of change going on in the world today. So if we’ve clearly defined what’s happening today it can help alleviate some of that, that change and hesitancy to it. If we have clearly defined processes and expectations of the people that that are in place today, as we start to think about the guardrails around it, there’s a couple of different lenses that I think about. The first one being, as Davis called out, is the maturity of the technology. So a lot of ML technology has been around for a long time. Activity capture is one of the things that we’re talking about. Some of the AI systems around to that they’ve been proven to work. And there’s a lot less hesitation there. Some of the newer things around like LLMs or ChatGPT and how we use them, those are the newer ones where there’s some concern around, what does this mean for the organization? So thinking again, back to your processes, how can you introduce those technologies to help alleviate some of that?
Risk is one way that we think about it here at CIQ. So we’ve started account planning as a new process. And I’m sure there’s a collective groan from everyone on the call, as I mentioned, the words account planning. But one of the big things around account planning is taking the time to understand your customer’s market and their company in a lot more detail. And our enterprise customers. That’s based on a lot of publicly available information using an LLM like ChatGPT to help you understand the market, the company’s position, the problems that they’re solving, which is the foundation and base of your account plan, which does not require any of your proprietary own information from CIQ, is a low-risk augmentation to a process that exists, and leveraging a tool like AI to start to introduce it. Now, as we’re talking about starting to leverage our own proprietary information, you then want to be thinking about things that have been proven in the market, like an outreach, like a Gong. Big players, established companies that have put their technologies out there have been proven, and that helps to reduce the risk of introducing some of those bumpers and guardrails that you talked about. Mark.
Mark Schopmeyer : A lot of great points there. Maybe one last quick question then I’d love to jump into the next case study or use case, but I’m sure a lot of sellers are on this call right now and question they’re asking. I’m hearing a lot of it. Automating my job. Is there a limit to the AI sales system of what it can do, or is it the intention to replace me entirely?
Davis Giedt: I could jump in with it. I guess a quick answer there. It’s really the way we’re viewing it is, and for the foreseeable future and probably beyond, is it’s truly an augmentation. A positive augmentation, hopefully, to what a sales rep does on a daily basis or on a weekly basis. There are no indications that AI is going to be able to replace a B2B sales reps job in today’s world. As far as what we’ve seen in the marketplace in a lot of cases. There was a study from MIT that came out a few weeks ago. And part of what they’re talking about in that study was just the cost implications of how expensive it is to try and implement some of these AI solutions. This goes beyond just goes beyond just sales. It’s across all different business applications. But it’s a huge factor when you think about if you’re investing in an AI tool, you’re trying to help sales reps be more efficient. Ultimately, as Andrew said earlier, you’re trying to drive to a better expense-to-bookings ratio in your organization. The cost of implementing a huge LLM type of solution in your organization, the risks that come with it and whatnot may outweigh the actual potential benefit of it, versus just using human intervention to get the job done. So that combined with just sort of the sophistication or lack of sophistication of a lot of the tools today are leading many organizations to go down the path of augmentation, productivity uplift, as opposed to sort of replacement.
Mark Schopmeyer : Great point.
Andrew Morales: And Mark, I actually love your take on this as you think about the space because I know that you and Conway are thinking about how this gets introduced to CIQ and our platform, which is wrapped in customer-facing as well. But I agree with Davis in terms of the augmentation, I think about it a lot in terms of some of the things like Excel as a tool has augmented my workflow so that I don’t need to use a calculator on a day-to-day basis. And by the way, I’m terrible at math as well. So it’s really been helpful. And what it allows us to do is then focus on those higher-value activities in terms of spending time in front of a customer, instead of me chasing an AE or BDR to enter information into a CRM. They don’t have to spend the time to do that anymore, and they can take their time and spend them on those higher-order, higher-value activities, which are more nuanced in today’s in today’s space. And there isn’t the contextualization around some of the LLMs that exist to be able to move those things forward. So I really do see it as an augmentation. And that’s where we’re seeing a lot of the benefits in today’s workflows and what we’re planning on from a future state standpoint as well.
Mark Schopmeyer : I think you guys both hit on points correctly. And even how we think about tick, a lot of it’s been around augmentation. It’s automated insights. It’s like, tell me, give me information more at my fingertips and put the bumper rails. And I think that’s a good principle. The analogy I think about it is take autonomous driving a space that has billions, tens of billions of dollars of capital running through it. Everyone knows the holy grail, automated autonomous driving. You’ve got like the next hundred billion-dollar company. Now what’s wrong? It’s a very, very hard problem. Like, everyone has the right incentive to drive into it. But you’re seeing today there’s actually a pullback. A lot of companies are realizing this is really hard to do the full thing. But if you drive any modern car right now, you’re seeing augmentation. You’re seeing like, hey, this is what we can do. We’re going to put some parallel driving or whatever it is, like the easy stuff to make drivers just better. And I think that’s where we are today. And I think that’s where we’re going to see for the next probably decade or if not more. Well, great, great conversation. We’d love to jump to the next one. Boosting customer experience through AI-powered service interactions. Davis, you spent time around this space. Why does this matter? And how is AI going to help around this?
Davis Giedt: Yeah. Before we get into the specifics of how, what I mentioned earlier, how service organizations, customer service organizations are leveraging AI, let’s just take a step back and think about the broader point of service organizations. And it’s really to drive better customer experience, better customer success, higher renewal rates, resale rates, better net Promoter score, etc. Those are all sort of the outcomes and ways that I help organizations do that is they help organizations align customer segments with a level of service. So as these are also just sort of broad goals of a service organization. So thinking about do I really need a human to interact with a customer at the lower levels? Lower purchase levels, lower touch levels, or is there a way that a chatbot can actually drive towards a better customer experience? Other ones are in the world of enabling service reps and technical service reps and whatnot. There are a lot of powerful ways that I can enable them. Just similar to what we talked about in terms of sales assistants is offering insights to them based on past interactions and positive interactions. What we’ve seen a lot of powerful sort of quick advancements in the service industry particularly around this, the service world.
It’s probably been one of the more advanced LLM implementations that we’ve seen over the past year or so. A lot of companies are coming to us asking for consulting advice on how to drive costs out of the service organization today because it’s a huge cost center. They’re seeing big opportunities for automation and whatnot. Not surprisingly, some results from a customer service survey that we did of customer service organizations last year found that in some cases, 30 to 40% of a customer service reps time was spent on pretty wrote activities. So answering two questions: one where is my order? And two is can I reorder low-cost or simple product A, B or C? So these are solutions that you would think can be automated pretty quickly in driving costs out of the organization. But it’s not always that simple. In a lot of cases, you can automate and put in an AI-powered, LLM-driven service bot to sort of qualify the service interaction, ultimately get organizations or get buyers to purchase products or repurchase products, give an update on their order.
Kind of like you picking up the phone and calling a B2C organization with some of the same questions. But in other cases, you need that chatbot or that capability to be able to route the potential buyer to the right place. So they’re getting sort of connected with the right rep, whether it be a technical service rep or a customer service rep, to resolve their issue. And the way that organizations are doing that is they’re taking thousands or hundreds of thousands of historically resolved customer interactions or unresolved customer interactions, loading them into a large language model and a machine learning model, and helping that model to or training that model to understand what types of buyers, which types of inquiries, how quickly they’re resolved, what are the fastest paths to resolution. So all these different propensity to resolve type of metrics and propensity to resolve type of indications go into that, which ultimately feeds not only the true self-service interaction, but also the ability to guide a service rep, much like you would a sales assistant towards the right resolution with the customer.
Mark Schopmeyer : I see a quick clarification question from Allison Carter. Are we talking about SaaS or services? But I think you’re talking about in general, just the service interaction with customers and how these tools are being applied in, whether it’s a SaaS offering will most likely be a SaaS offering and enabling the service industry.
Davis Giedt: That’s right. Yeah, exactly. So, a good example might be an organization that is selling building materials, just to keep it simple, selling building materials to contractors. And so a contractor might be used to picking up the phone and reordering doors or windows on a regular basis, which actually requires a lot of time and cost from a service rep. There’s ways to automate that and drive the customer towards a self-service module driven by an AI chatbot that would ultimately get them to a good outcome. There are also situations where there might be a combination of these basic service level interactions, as well as more complicated technical interactions, like in an organization selling life sciences and analytical instruments products to universities. There could be situations where people are trying to reorder basic products like reagents or beakers, and there could be other situations where they need to talk to somebody. They’re a PhD level scientist, and they need to talk to somebody about a very technical issue. And an AI tool can help to qualify those different interactions and drive them to the right place quickly, and ultimately driving costs out of the organization so that a human doesn’t actually have to do the qualification of that interaction.
Mark Schopmeyer : Perfect. Well, maybe Andrew and or Davis, would love to hear your take on what you guys have seen so far in practice. Maybe. Davis, what are you seeing so far? And some of the things that you’re seeing as applied to.
Davis Giedt: Yeah. I mean, I think I offered a couple of them already in terms of the ability to train the model and ultimately try and drive less reliance on the customer time. We’re also seeing that organizations are investing in trying to reduce service service rep ramp times with personalized, interactive training. So there are AI tools, much like what you see in the sales world or in the marketing world that help to train sellers faster. So they’re identifying minimum service skills necessary before considering a service rep to be fully ramped. And sometimes they get down to the sort of personality traits and interests they offer interactive exercises to keep service reps skills honed as they grow in their careers. To help them optimize information uptake. They also can feed information back to management, service management to understand sort of what the opportunities are for improvement from a training standpoint. Another one that we’ve seen thinking more broadly about customer experience tracking and voice of the customer tracking from a service world is helping to design better customer feedback surveys. So there are AI tools that can help, to come in and look at a survey draft or even start to develop a survey draft for organizations that are more likely based on their aggregation of a bunch of different organizations, outcome and response rate and whatnot. Help design questions and write questions that actually get a better response rate and more insightful responses to these customer feedback surveys, which are always a challenge for many organizations.
Mark Schopmeyer : It’s kind of the way I kind of look at this is I probably would proactively share, I think the customer service journey for a lot of companies, especially consumer-oriented ones, is a really crappy one. I think in the name of automation and cost savings, the service experience is degraded massively. So I look at this as hopefully with these tools, we’re at a rock bottom of what we would expect for service quality, and we should actually see quite a bigger lift if we get the right answers sooner and faster. Andrew, what’s your take on this? What experiences or examples have you seen out there?
Andrew Morales: Yeah. So I think a lot about our go-to-market motions. And in a lot of what I’ve seen is augmentation of internal workflows is where a lot of the technology is kind of today and how I’ve seen it employed in previous lives. But one of the ways we’re employing this here at CIQ is we’re starting to spend a lot more time as we move into FY 24, really talking about our customers and what’s our customer experience, what’s the totality of the customer journey and experience. And internally, the way that we’re starting to put that into practice is we’re using an engagement model. The Alexander Group has written quite a bit about it. ILAER: identify land, adopt, expand and renew, which is our internal process. And there’s a common theme here, Mark, people, process, technology that I’m going to keep the drum beat on. But we’re starting to define what are the processes internally that we want to support our entire customer journey and experience with, so that we provide a good service experience for our customers. And the way that we’re doing that today is within our customer interactions of our website, is using things like bots, whether they’re AI or chat bots, to help point customers to the right part of the website so they can get the right resources at the time that they need, the resources for what they’re trying to do, whether they’re educating themselves, whether they’re trying to identify specific part of our product or learn more about how we can augment their workflows.
We’re then doubling down on that by using similar bots and technologies to route people to the person at the point in time that they might need to talk to somebody. So if one of our potential customers or customers comes in and wants to talk with one of our go-to-market team members, we’ve defined our internal processes and that’s something that we want to support with SLAs. And we’re using this technology then to appropriately get somebody in real time talking to our customers to provide a fantastic customer experience and the service at the time that they want it at the level that they’re expecting. And we’re doing that without having to increase the resources as an organization from a human perspective because we’re leveraging the AI technology to point people to the right direction and connect them with the right people at the point in time that they want to be connected to them. So it goes back to defining those processes that are going to delight your customers to deliver a fantastic customer experience through that entire customer journey, whether it’s reordering beakers or products on the back end or somebody trying to educate themselves about your space, your market, and what it is that you do to help them out.
Mark Schopmeyer : Yeah, I think I hear this from our customers, I hear from prospects, I hear it from just people in the industry where a poorly designed chatbot, the one that we are familiar with today on a website leads to a poor experience because it forces a form. Fill out all that stuff. And people just want to get a simple answer. And I think what this does, similar to your point, Dave, is I think it just elevates the experience. You don’t have to now rely on a very properly designed workflow. It now can feed all the information appropriately, while still getting all the relevant information to help with the experience. So awesome. Very cool. We’d love to jump to our last big topic. I know it’s one a lot of people have been pinging me about that. They just want to hear a lot more perspectives around it. I think there’s a lot of applicable use cases around this and maybe turn it to you, Davis, is why does this matter? Why are you seeing AI in this?
Davis Giedt: Yeah. I mean, I always go back to the tenets of a RevOps organization, which it’s best to define RevOps for a lot of folks because it’s not always clear. In our world, at Alexander Group, we define RevOps as the sort of organization or in some cases, it’s a group of organizations, that cover marketing, sales and service operations. So everything from figuring out how to run the marketing organization, not from a leadership standpoint, but from an enablement and tracking and operations standpoint to everything in sales ops, which tends to be the biggest chunk of RevOps. So that’s everything from segmentation and targeting down through designing job roles and coverage models, setting quotas, setting compensation, enabling channel partners, managing sales technologies, then everything in the service operations world as well. So helping service reps be most successful, tracking service interactions and call resolution times and all the metrics that go along with it and delivering tools to them. So anyway, it’s a pretty broad definition, but there’s some tenets that always matter across the organization. A few of them are enabling decision-making with clean, accurate data. And there’s a ton of ways that I can support that, improving insight, sharing across departments or across marketing, self-service with the wide sort of remit of RevOps, it’s really important that happens.
Tying KPIs to strategic goals and shareholder expectations is also really important. There’s some AI tools that help organizations figure out how to which KPIs to prioritize, which ultimately lead to more strategic sort of outcomes and goals, whether it’s net revenue retention or profitability or both, or expense to bookings. There are some AI tools that have a sophisticated capability to do that. And then ultimately, the sort of end all be all is figuring out how to drive a better customer experience. And there’s again, we’ve talked about a ton of AI tools that are focused on that. But there are a lot of ways that RevOps can support that as well. More specifically, this all starts. It always starts with data. In any RevOps structure, the cornerstone of the bedrock of everything is good data. And what we’ve seen is there are a lot of great ways that AI is augmenting the RevOps ability to capture and replace, clean, organize, analyze, etc. the data that they need to deliver insights to the organization.
Mark Schopmeyer : Yeah. I think there’s a lot of potential there. It almost sounds like a cheat code if it can really solve all these things. Andrew, I would love to get your take on the practitioner’s lens around this. How are you seeing all this come together?
Andrew Morales: Yeah, I would say that a lot of RevOps. And as Davis called out the starting point of it starts with a lot of sales operations kind of background and practitioners. And I know that we’ve got a lot of practitioners on the call today that are interested in this topic. And one of the conversations that really is starting to drive some momentum, whether it’s on various forums or LinkedIn or articles is an idea of around strategic RevOps. And I think one of the things that Davis called out, which resonates with me and how I’ve seen it play out here in various lives previously, is that this idea of RevOps moving from just beyond managing the tech stack to helping connect the dots between the strategic plan and direction of the organization, where it’s going and the execution of that plan. And so how do we build OKRs or measurements or KPIs to make sure that we’re moving those things forward and actually driving the execution within the business? And the way that I’ve seen successfully, individuals and organizations jump that gap from just owning and managing the tech stack to becoming partners in helping drive the execution of that plan is by bringing insights to the table about what’s working, what’s not working around the execution of the plan.
And to do that, as Davis called out, you need to be able to leverage data at scale to make data-informed and data or data-driven decisions about not just what’s working and what’s not, but what direction should we be going and what is going to pay off for us in the end. And so when I think about the augmentation of our workflows, there are a number of them that exist. A core practice of a RevOps organization is managing a forecast, for example. So we go back to talking about activity capture within an organization. Are you designing the processes and employing the right technologies to automate activity capture so that you can run an accurate forecast call, build a predictable and accurate forecast because all that is based on data? And so are you designing the right processes that exist? But in the spirit of talking about very tactically, how do we walk away from this conversation? With things that maybe some of our practitioners can use? I’d like to focus a little bit more on a recent example. As we went through FY 2024 planning and how we went through an exercise of reducing the world of focus of our account executives and our BDR organization to where we believe we are actually going to win and win at a higher likelihood.
And a lot of individuals on the call, I’m sure, are familiar with things like account tiering, account prioritization. And that’s something that we really took to heart this year as we built our FY 24 plan. It previously, we assigned every account out in our database, which was about 115,000 accounts to all of our account executives. And they’re essentially looking for opportunities in the entire world of accounts that exist today. And what we know is that focus is going to drive higher win rates and outcomes for the team. So how do we actually focus that attention? And to do that, we built an account tiering model to help build books of business to create focus for our teams. Now, we are not an extremely large RevOps organization here. And so to what Davis had talked about in his article and what we’ve been talking about today is how can I augment the workflows that we’re doing? And we were able to leverage ChatGPT and LLMs to help with some data cleanup. And back to the bumpers and guardrails that you called out, Mark, not providing proprietary data, but helping us to create industry groupings.
Industries are set data points that exist. Zoominfo returns a number of different data points around how many industries, sub industries, and we wanted to aggregate those into specific industry groupings that we thought we could win best at, and using a tool like ChatGPT to help us clean up non-proprietary data, put us in a spot that within less than a week in a really small RevOps team, we were able to create a really robust account sharing model that reduced the world of accounts that are assigned to our eyes by 99%. We’ve reduced the world of accounts assigned out to our entire account executive team by 99%, and we believe our win rates are going to improve as a result of that because we have our reps focusing on the accounts that are going to drive the most outcome and revenue for us and at higher win rates as well. And so that’s a really tactical example of how we use AI and an LLM to help augment our internal workflow. And back to the discussion around guardrails and bumpers. We did that without using proprietary data because we’re using it to augment the manual workload. That would typically take weeks on end to clean up before we could even get into an account tiering model build.
Mark Schopmeyer : But this is pretty, pretty huge applications. And what I’m hearing from you today is we’re already starting to do a lot of this work today, so hopefully everyone can take on the call. This isn’t a future technology, and we have to wait for 1 or 2 years for it to really be applied. There are some things that are low-hanging fruit that can be applied today, that you’re going to see some pretty massive savings around.
I know doing a quick time check, I know we’re coming up too short on time, and I want to just make sure we’re getting some questions from the audience. There’s a quick reminder. There’s a Q&A part of this module in Zoom. Please drop in your questions there. I do see a question from Brendan Powers. Curious how you are routing customers to the right resource. Is this through an actual in-house built routing logic, etc.?
Davis Giedt: Yeah. Maybe I can jump in to start. I think it depends on the use case. If we’re talking about whether this is a service interaction or if it’s a new potential customer that’s coming in at the top of the funnel, etc. but this again, I think it comes back to data and the ability to profile customers. There are a lot of organizations that have invested really heavily in their ability to sort of drive intent. So this is what we mean by intent is tracking everything that a potential buyer or suspect, if you will, is doing in this sort of web world, digital world, whether it’s interacting with your website or interacting with forums or social media or anything about your brand or related to your brand, and then profiling that customer and ultimately filtering them down to the right resource, whether it be a BDR or an SCR or just a web form to sort of help qualify that lead. It really depends on the profile and the amount of information and data that you have at your fingertips to be able to manage that interaction. Again, it also has to do with the scale and sophistication of the operation.
Are we talking about thousands of leads per day or hundreds of thousands per week? Are we talking about a handful per week in a situation where you’re selling large deals or you’re a smaller organization, etc., and you don’t deal with that many leads, you may not need to invest huge dollars in the capability to do that in the situation where you’re managing tons and tons of leads, there’s a lot of variability in them. It may be really important for you to invest in that kind of capability. But to answer your question more specifically, there are certainly tools that support that support this. There is in-house built routing logic. There’s both. There are different sort of approaches and philosophies on build versus buy. What we typically see is it’s a combination. There’s a combination of you’re using a tool that helps get you 50% of the way there, and then you’re configuring or building on top of it of the rest of it to get you to the sort of finish line that of something that actually works in your organization. And then, not surprisingly, when you take that approach, there’s typically better adoption internally of the organization of the actual tool.
Mark Schopmeyer : Andrew, anything you want to add? I saw you were about to jump in.
Andrew Morales: I think Davis hit all the points. We’re using a combination internally. I think if the question is directed specifically at us. And so as Davis talked about, there’s technologies and tools that can provide some AI augmentation to to routing, but it’s dependent on having data to be able to understand and and make recommendations. And so the internal routing logic helps us establish what is the baseline. So the data capture exists. And then recommendations can be made on top of it. So it’s a combination of the two.
Mark Schopmeyer : Let’s see. One more final question. And this one’s maybe a quicker one. What if everyone on my team is not on board with using AI at this point? How could I get them more comfortable and start using it regularly?
Andrew Morales: I love that question. And I think it applies to a lot of our practitioners on the call today. From a RevOps standpoint, whenever we introduce change or new technology, it can be a bit of a friction point. And I remember the first time I rolled out a sales engagement platform, a lot of our legacy and tenured sellers were really resistant to the change, that this was not going to help them, that this was not going to benefit them. And you absolutely have some of those rock stars and superstar performers that are going to be resistant to change. I would say that a best practice that I’ve used and that I’ve seen successfully used is it goes back to the framework we were talking about. Do we have clearly defined processes in which these tools are going to step in to help augment the workflow? And if we do, introducing them in that capacity can help you prove the value in the use case of why it’s important to take the leap of faith and adopt something like this, regardless of how tenured a seller is going to be. If you can articulate that their win rate, reply rate, whatever it might be, time to retire their quota is going to improve by a factor of with use cases to demonstrate that. That’s how I’ve seen successfully bringing people along the change journey to say, yeah, let me give this a shot. It goes back to being able to clearly define the process, how it’s going to help, and then being able to articulate stories, where we’ve seen this win successfully so that people take a chance at trying that out.
Mark Schopmeyer : It’s a great call out. There’s something that I’ve seen and I’ve learned from an enablement practitioner when they’re giving me advice. But you kind of need to show people along the journey. Make sure they’re part of it. I think the more forceful the change is, the more resistance you’re going to see on the flip side of it. So, all things are called out. Customers, the stories, the metrics, etc., but your best bet, I think also, personally, I think starting with some of your earlier, younger, tenured employees are going to be faster adopters than those who have been set in their ways, built years and years of habits. It’s just going to, I think the practicality is there’s a lot more resistance for people who have built years of habits and feel like, hey, this is what’s working for me. I just want to keep it simple. It’s going to be a change over time. It’s not going to be a rapid one.
So with that said, I think we’re coming up on time. And I wanted to first appreciate you both. Thank you for taking time out of your day to share a lot of your great insights. From both the research to the practitioner side, and thank you to the audience for participating, asking great questions. That wraps up for today’s webinar. Please follow Captivate IQ and Alexander Group on LinkedIn. If you want to learn more about these types of events, we will send out an email shortly with a recording of today’s session. If there are more questions, or you’d like to hear us talk about the other two use cases that we didn’t cover today, happy to follow up and schedule another session. So again, thank you all for the time and have a great rest of your Tuesday.
Davis Giedt: Thank you.
Andrew Morales: Thank you.